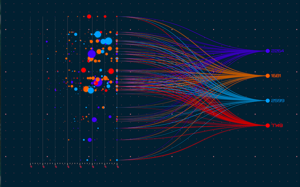
ML and Business Strategy
There are no doubt numerous techniques in which data can be collected, and even more numerous data sources which store the data itself. However, once data engineers have extracted, transformed and loaded this data into a data store it needs to be analysed. Many companies are choosing to use artificial intelligence and machine learning (ML) to glean insights from their data. The insights supplement decision making or, in some cases, completely automate the decision making process. There are a couple of examples of this: in the healthcare industry triage process, machine learning is used to categorise and prioritise incoming patients. Providing nursing staff with this technology helps increase diagnostic and therapeutic assessments and also enables remote triaging. The most frequently used machine learning models used here are based on XGBoost and Deep Neural Networks; other models have been considered such as Logistic Regression but the performance decreases. One of the main industries that uses ML is financial services. Here, ML is increasingly used by companies to aid decision making and automation of processes. According to the Bank of England, 72% of UK financial services firms are developing machine learning applications, with the insurance and banking sectors setting the pace for absolute usage. Despite this, firms are aware of the constraints of machine learning deployment that arise due to the Prudential Regulation Authority’s and Financial Conduct Authority’s existing regulations lacking clarity. An important point to raise here is that despite this lack of clarity, regulatory authorities need to ensure regulations ensure safe and responsible adoption of machine learning. Within FS, the main business areas in which ML is deployed include customer engagement and risk management and compliance. Customer engagement has the highest percentage of post deployment applications and is seen at various stages throughout the customer lifecycle. There are many other business ares which use it but these are not within in the scope of this blog. Firms deploy ML applications for a variety of uses, however, they can be both from internal and external implementation. They can be externally implemented by third party vendors or co-implemented with third party vendors providing any other the services in the vertical integration. This may be cloud storage, the ML models, software packages or data input. These applications need to monitored and tested to validate performance. The most commonly used methods are outcome monitoring against a benchmark. Here, performance and outputs of the model are compared against historical data. The historical data used will vary depending on the business area or industry but is most commonly profitability, customer satisfaction or pricing. The next most common method is data-quality validation: this is used to detect errors, biases and risks in the data. Once model performance is validated and models are deployed results can be used to aid decision-making. Typically in financial services, ML models are most commonly used in pricing and underwriting, with complex models used for credit pricing and insurance underwriting. These models are at an advanced stage of deployment and are used in expected loss accounting, claims accounting, motoring for insider trading or market manipulation, directing queries within customer interfaces, compliance/AML/KYC checks, trading strategy and execution and payments authorisation. ML has a variety of use cases and is being adopted by more and more firms, not just in financial services but also in other industries such as healthcare. Most business use it to aid in customer engagement, with it being used to classify, predict or optimise data based on customer behaviour, with this in turn used in strategic decision-making. Businesses will no doubt continue to deploy more complex ML models and as these processes become more common and understood they will trickle down to smaller firms.